What is Generative AI?
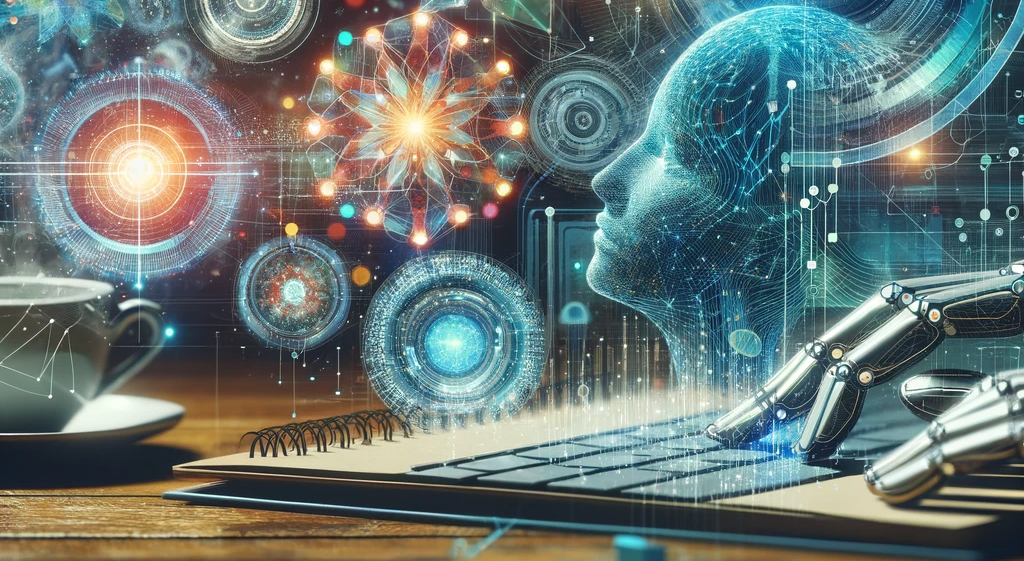
Generative AI is a fascinating subset of artificial intelligence focused on creating new, original content. Unlike other AI types that analyse or process data, generative AI generates new data that is similar but not identical to what it was trained on.
In a world where technology is increasingly intertwined with our daily lives, Artificial Intelligence (AI) stands as a cornerstone of modern innovation. At its core, AI is the simulation of human intelligence processes by machines, particularly computer systems. It’s a multidisciplinary field that blends computer science, cognitive science, linguistics, psychology, and more, aiming to create machines capable of performing tasks that typically require human intelligence.
This fascinating realm of technology encompasses various functions such as learning, reasoning, problem-solving, perception, and language understanding. AI systems are designed to interpret complex data, whether text, images, or sounds, learn from such data, and then make decisions or predictions based on this learning.
From virtual assistants on our smartphones to sophisticated robotics in manufacturing, AI is reshaping the way we live and work. Its applications range from simple tasks like filtering spam emails to more complex ones like diagnosing diseases, driving autonomous vehicles, or providing personalized education.
At its best, AI promises to enhance human capabilities, streamline tedious tasks, and solve some of the most complex challenges facing our world. However, it also raises important ethical and societal questions about privacy, security, and the future of work. As AI continues to evolve, it invites us to reimagine the boundaries of what is possible, urging us to consider both its immense potential and the responsibilities that come with it.
Types of AI
Artificial Intelligence (AI) encompasses a wide range of technologies and approaches, each with its own unique capabilities and applications. The various subsets of AI can be broadly categorised based on their functionalities and methodologies. Here are some of the key subsets:
- Machine Learning (ML):
- This involves algorithms that enable computers to learn from and make predictions or decisions based on data. It’s a core part of AI where systems improve their performance with experience.
- Deep Learning:
- A subset of machine learning, deep learning uses neural networks with many layers (hence “deep”) to analyze various factors in large amounts of data. It’s particularly effective for complex tasks like image and speech recognition.
- Natural Language Processing (NLP):
- NLP focuses on enabling machines to understand and interpret human language. This subset of AI is behind technologies like language translation services, chatbots, and voice assistants.
- Computer Vision:
- This involves teaching machines to interpret and understand the visual world. Using digital images from cameras and videos and deep learning, machines can accurately identify and classify objects, and then react to what they “see.”
- Robotics:
- Robotics involves the design and creation of robots, which are programmed to perform tasks. When combined with AI, these robots can perform complex, intelligent tasks.
- Expert Systems:
- These are AI systems that use knowledge-based databases to simulate the expertise of a human in a specific field, providing advice, reasoning solutions, or clarifying uncertainties.
- Reinforcement Learning:
- This is a type of machine learning where an algorithm learns to make decisions by performing actions and receiving feedback from those actions, essentially learning from trial and error.
- Generative AI:
- This subset of AI focuses on creating new content, such as text, images, and music, that is similar to but distinct from the data it was trained on.
- Predictive Analytics:
- This uses statistical algorithms and machine learning techniques to identify the likelihood of future outcomes based on historical data.
- Emotion AI:
- Also known as affective computing, this area of AI involves building systems that can recognize, interpret, process, and simulate human emotions.
- Cognitive Computing:
- This subset aims to mimic human thought processes in a computerized model, using self-learning algorithms that use data mining, pattern recognition, and natural language processing.
Each of these subsets plays a crucial role in the broader field of AI, contributing to advancements in various industries and sectors. They often overlap and complement each other, creating systems that are more intelligent and capable of handling complex tasks.
Generative AI
Generative AI is a fascinating subset of artificial intelligence focused on creating new, original content. Unlike other AI types that analyse or process data, generative AI generates new data that is similar but not identical to what it was trained on.
Generative AI models, such as Generative Adversarial Networks (GANs) and Variational Autoencoders (VAEs), learn the underlying patterns and distributions in training data. They then use this knowledge to generate new, original content.
Generative AI can enhance creativity, offer new perspectives in design and art, and accelerate research and development processes in various industries.
Art and Images
AI can create realistic artwork and images that are hard to distinguish from those created by humans. For example, GANs have been used to create original paintings and digital art.
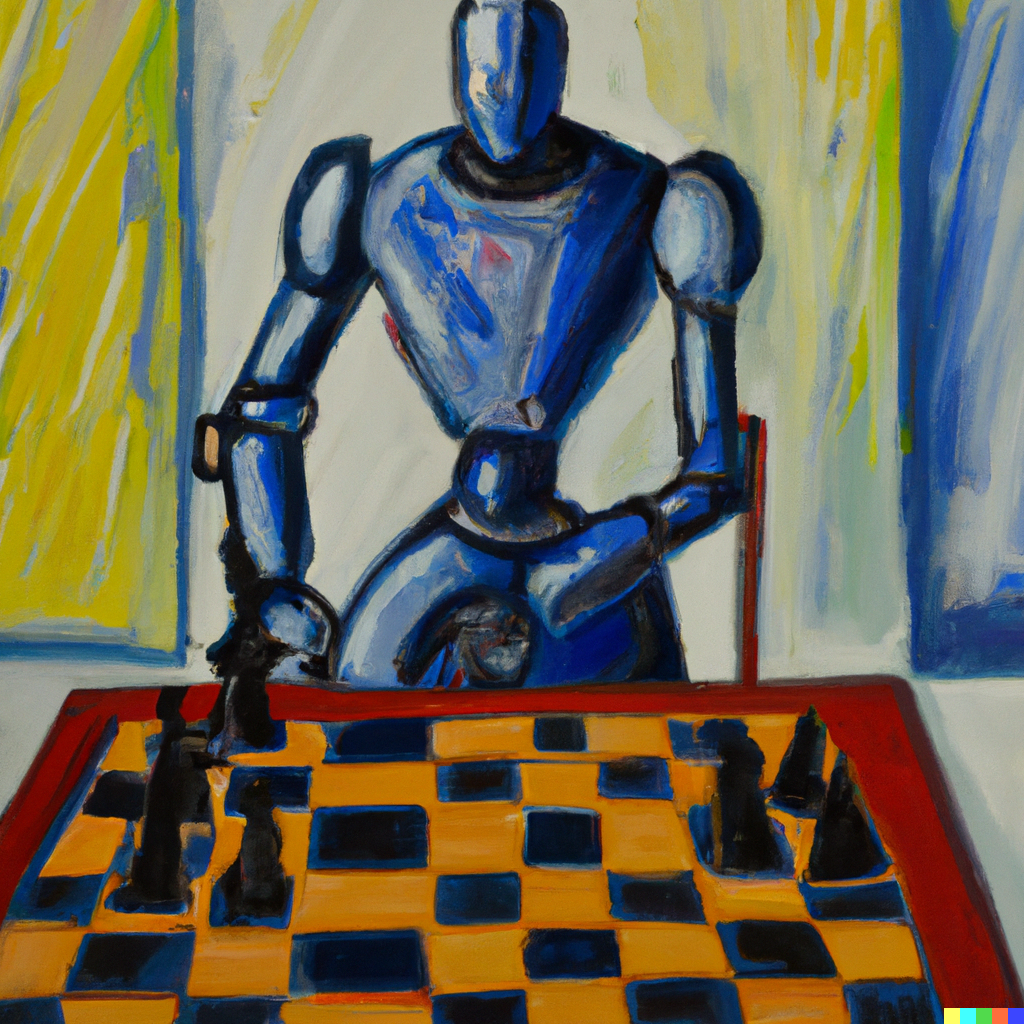
Music Composition
Generative models can compose music in various styles, creating original pieces that sound like they were composed by human musicians.
Text and Content Generation
AI can write articles, poems, or even entire books. Tools like GPT (Generative Pre-trained Transformer) are capable of generating coherent and contextually relevant text based on input prompts.
Product Design
In fields like architecture and engineering, AI can generate innovative design options for products, buildings, and machinery.
Drug Discovery
Generative models can predict the structure of new molecules for drug development, speeding up the process of finding new treatments.
What about the future?
In the rapidly evolving realm of technology, generative AI stands as a beacon of innovation, poised to redefine the boundaries of creativity and practical application. As we look towards the future, it’s exhilarating to envision a world where AI-generated art and music become seamlessly integrated into our cultural fabric, offering new forms of expression and artistic collaboration.
The potential of generative AI extends beyond the arts, promising to revolutionize industries by accelerating drug discovery, enabling the design of groundbreaking architectural marvels, and even personalizing educational content to adapt to individual learning styles. However, this journey is not without its challenges. Ethical considerations, particularly around the authenticity and originality of AI-generated content, will necessitate thoughtful navigation.
As we embrace this future, the fusion of human creativity with AI’s limitless potential could lead to a renaissance of innovation, reshaping our world in ways we have yet to fully imagine.